Explainable AI Techniques: Making AI Decisions Understandable
Discover explainable AI techniques that make AI decision-making transparent and understandable. Learn how these methods enhance trust and clarity in AI systems.
Explainable AI Techniques: Making AI Decisions Understandable
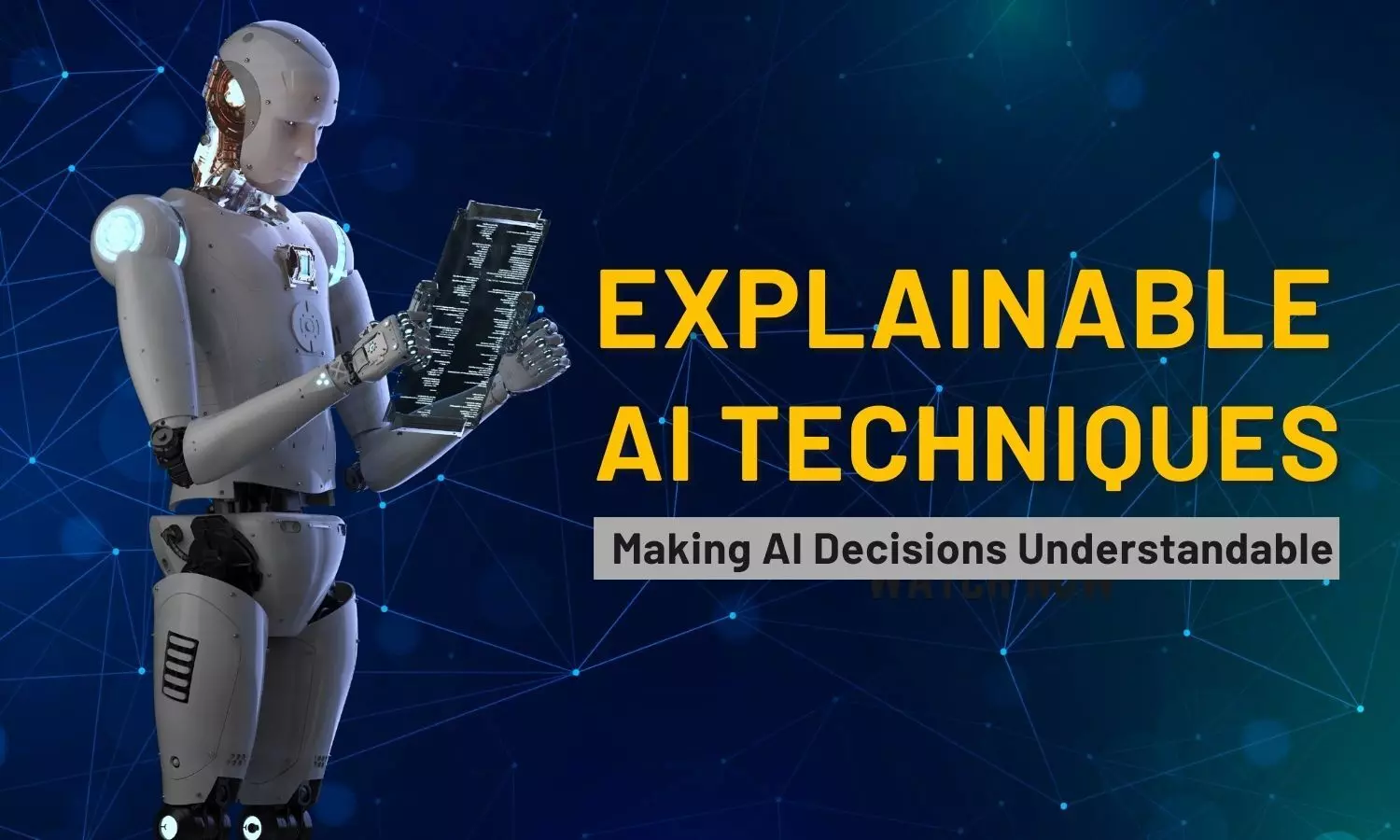
Explainable artificial intelligence (XAI) encompasses methods and processes that enable users to understand and trust the outcomes produced by machine learning algorithms. It involves describing an AI model, its anticipated impact, and any potential biases. XAI is essential for assessing model accuracy, fairness, transparency, and decision-making outcomes, and it is key to building trust and confidence in AI systems within an organisation.
As AI technologies advance, the complexity of algorithms often turns their workings into a “black box,” making it difficult to interpret how results are achieved. These black box models are built from data; even the engineers or data scientists who create them may not fully understand the internal workings or the basis for specific results.
Understanding how an AI system reaches its conclusions offers numerous benefits. It helps developers ensure the system functions as intended, meets regulatory standards, and allows individuals impacted by decisions to challenge or modify outcomes.
Explainable AI techniques are crucial for making AI decisions clear and enhancing transparency in machine learning. XAI models and methods elucidate how AI systems reach their conclusions, which is vital for fostering trust. Implementing XAI helps organisations ensure transparency, especially in high-impact areas like healthcare, finance, and law. Furthermore, XAI aids in regulatory compliance by offering detailed insights into AI operations, allowing businesses to adhere to legal standards while upholding fairness and accountability in AI processes.
How does Explainable AI work?
With explainable AI and interpretable machine learning, organisations can access the decision-making processes behind AI technology, enabling them to make informed adjustments. Explainable AI enhances the user experience by fostering trust in the AI's decisions, ensuring users feel confident that the system is making sound choices. It also addresses when AI systems provide enough confidence to rely on their decisions and how they can correct any errors that arise.
As AI technology advances, it's essential to maintain control and understanding of machine learning processes to ensure the accuracy of AI model outputs. This involves exploring the distinction between AI and explainable AI (XAI), the methods used to transform AI into XAI, and the differences between interpreting and explaining AI processes.
What distinguishes traditional AI from explainable AI (XAI)?
XAI uses specific techniques to ensure that each decision made during the machine learning process is traceable and explainable. In contrast, traditional AI often arrives at conclusions through machine learning algorithms without offering a clear understanding of how those results were achieved. This lack of transparency makes it difficult to verify accuracy and compromises the control, accountability, and audibility of AI systems.
Explainable AI Techniques
XAI techniques are built around three key methods: prediction accuracy, traceability, and decision understanding. These methods address both the technological and human aspects of AI systems, ensuring AI processes are transparent and reliable. Explainable AI, particularly in machine learning, is vital for helping users understand, trust, and manage AI systems effectively.
1. Prediction Accuracy: Accuracy is critical for evaluating the success of AI in practical applications. Prediction accuracy can be assessed by running simulations and comparing XAI output with training data. A popular technique for this is Local Interpretable Model-Agnostic Explanations (LIME), which clarifies the predictions made by machine learning algorithms.
2. Traceability: This technique ensures that AI decisions can be tracked and explained. For example, limiting the scope of machine learning rules and features helps create a traceable decision-making process. DeepLIFT (Deep Learning Important FeaTures) is an XAI technique that demonstrates the connection between each neuron’s activation and its reference neuron, providing a clear trace of how decisions are made.
3. Decision Understanding: This focuses on building trust by helping people understand how AI systems arrive at decisions. Educating teams on AI processes is essential for overcoming distrust and enabling effective collaboration between humans and AI systems.
Continuous Model Evaluation
Explainable AI enables businesses to troubleshoot and enhance model performance while helping stakeholders comprehend AI model behaviours. Monitoring key factors like deployment status, fairness, quality, and model drift is essential for scaling AI effectively.
Continuous model evaluation allows businesses to compare predictions, quantify risk, and optimise performance. The evaluation process becomes faster by visualising both positive and negative model behaviours alongside the data used for explanations. Leveraging data and AI platforms, teams can generate feature attributions for predictions and use interactive charts and exportable documents to visually investigate and analyse model behaviour.
Benefits of Explainable AI
1. Operationalise AI with Trust and Confidence: Build trust in AI models by ensuring they are interpretable and explainable. Quickly move AI models into production, simplifying model evaluation while enhancing transparency and traceability.
2. Accelerate AI Results: Systematically monitor and manage models to improve business outcomes. Continuously evaluate and refine model performance, enabling faster adjustments and more effective development efforts.
3. Reduce Risk and Governance Costs: Keep AI models transparent and compliant with regulatory and risk requirements. Minimise manual oversight, prevent costly errors and mitigate the risk of unintended biases in AI systems.
Five Key Considerations for Explainable AI
To achieve optimal outcomes with explainable AI, consider these important factors:
1. Fairness and Debiasing: Continuously manage and monitor fairness, scanning for potential biases in your AI deployment.
2. Model Drift Mitigation: Regularly analyse your model’s performance, recommending the most logical outcomes and alerting when models deviate from their intended results.
3. Model Risk Management: Quantify and mitigate risks by receiving alerts when a model underperforms. Understand the reasons behind persistent deviations.
4. Lifecycle Automation: Integrate data and AI services to build, run, and manage models. Use a unified platform to monitor models and outcomes & explain machine learning model dependencies.
5. Multicloud-Ready: Ensure AI projects can be deployed across hybrid environments—public clouds, private clouds, and on-premises—while promoting trust and confidence with explainable AI.
Use Cases for Explainable AI
1. Healthcare: Explainable AI can enhance diagnostics, image analysis, and medical resource optimisation. It can also improve transparency in patient care decision-making and streamline the pharmaceutical approval process.
2. Financial Services: Explainable AI can create a more transparent loan and credit approval process, enhance customer experiences, and expedite credit risk, wealth management, and financial crime assessments. It can also boost confidence in pricing, product recommendations, and investment services while efficiently resolving potential complaints.
3. Criminal Justice: Explainable AI can optimise predictions and risk assessments, accelerate DNA analysis, streamline prison population management, and enhance crime forecasting, all while detecting biases in training data and algorithms.
In conclusion, explainable AI (XAI) is essential for bridging the gap between intricate AI systems and human comprehension. By adopting XAI techniques, organisations can enhance transparency, build user trust, and ensure accountability in AI decision-making. This not only strengthens the reliability of AI systems but also aids in regulatory compliance by clarifying decision processes. As AI evolves, prioritising explainability will be vital for integrating AI across various sectors responsibly and transparently. Embracing XAI is key to fostering a responsible, ethical approach to AI that aligns with both societal values and regulatory standards.